
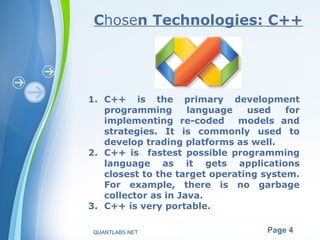
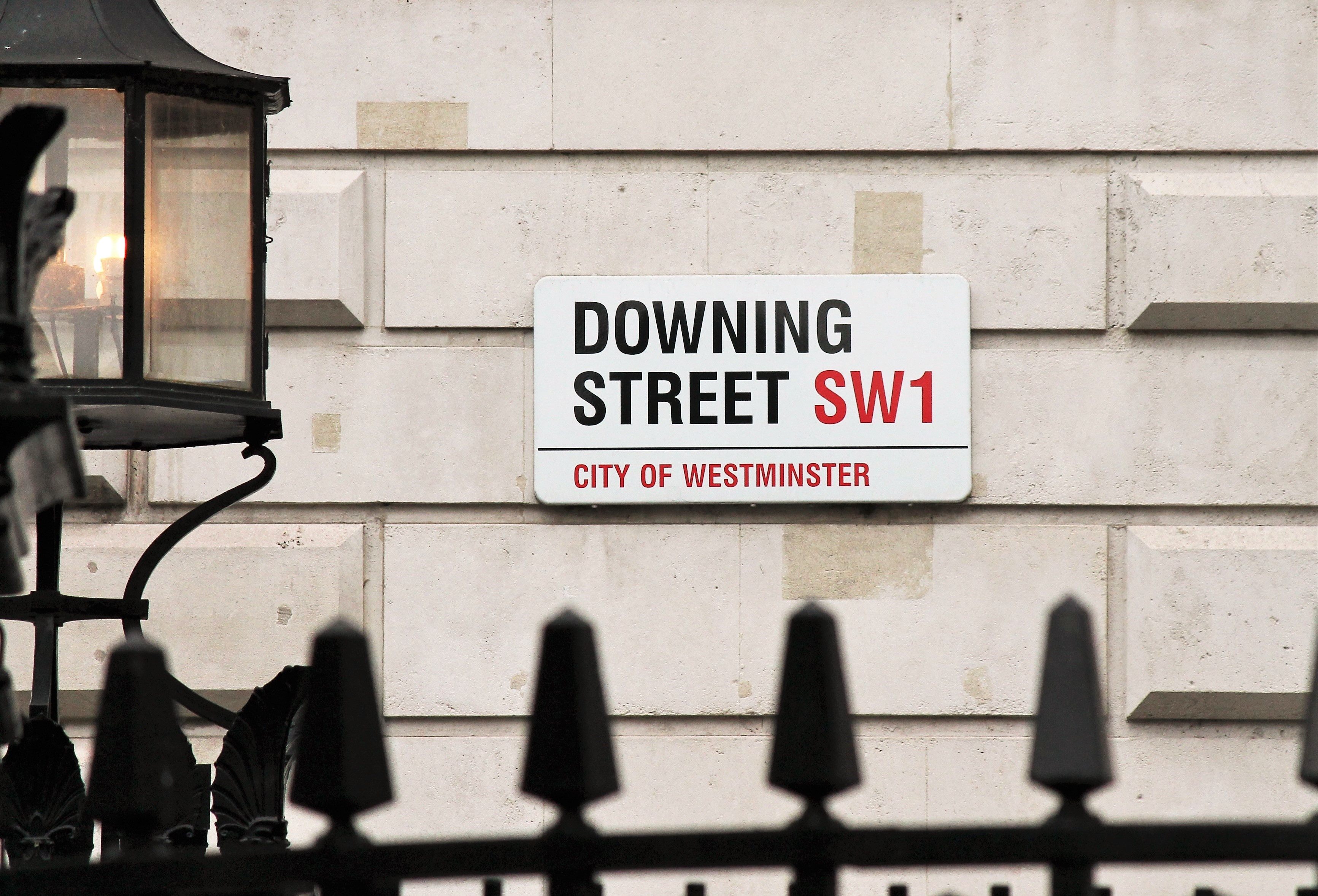
Continuous visual features, such as colour, orientation or location, are encoded in neural activity using population codes. In addition to the main methods, this toolbox contains a number of helper functions that may be useful for working with circular variables. In this case, the orientation values need to be multiplied by 2 so that the range covers the unique angles only, e.g. If the task involves reporting the remembered orientation of a bar, for example, the range of unique angles covers 180° ( π radians) rather than 360° (2 π radians).
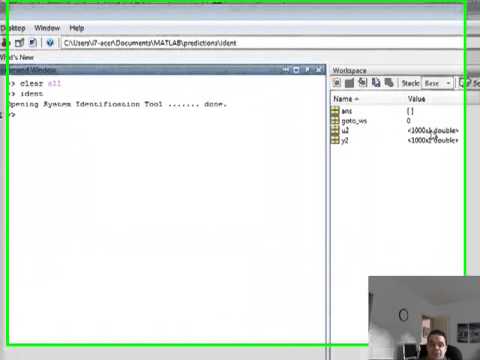
There is the possibility of confusion with tasks testing recall of orientations. Will ensure the resulting error measure is still in the range.
#DOWNIMG MATLAB TRIAL#
the error on a trial can be calculated as the deviation between the response value X and the target value T: The wrap function can also be used when adding or subtracting circular values, e.g. Where the wrap function constrains the resulting response values to the required range. Values coded in degrees can be converted to radians as follows:

The functions in this toolbox require inputs (stimulus feature and response values) to be specified in radians, in the range. The colour of each pixel shows the activity level of neurons whose preferred stimulus (the pairing of colour and location that activates them most strongly) is indicated by the position of the pixel on x and y scales. 2B illustrates how a population of idealized neurons could encode the information in Fig. Visual areas of the brain are known to represent these kind of stimulus features using population codes. In this case the item locations are chosen from an invisible circle, so all the information in the sample array relevant to the task can be described as a set of points, one for each item, in a 2D space with one axis indicating a colour on the colour wheel and the other a radial location. An observer with no memory for location would be equally likely to report any of the colours in the sample array.
#DOWNIMG MATLAB CODE#
If you refer to this code directly, please also include the URL of this webpage, or our homepage .Īn important aspect of tasks like this one is that accurate performance requires memory not only for colour (the report dimension) but also for location (the cue dimension).
#DOWNIMG MATLAB FREE#
The code is released under a GNU General Public License: feel free to use and adapt these functions as you like, but credit should be given if they contribute to your work by citing appropriate references from the above. This method avoids biases inherent in the mixture model approach, but also requires more data than the parametric methods. The purpose of this code is to provide estimates of swap frequency and response-dimension variability while making the fewest possible assumptions about the parametric form of the error distribution. Refs: Bays, Catalao & Husain, J Vis (2009) Schneegans & Bays, Cortex (2016).ģ. Responses are described by a probabilistic mixture of errors centred on the target feature, centred on one of the non-target features, or distributed uniformly across the response space. This older code fits a descriptive model to data from a single set size. Refs: Bays, J Neurosci (2014) Schneegans & Bays, J Neurosci (2017) Schneegans, Taylor & Bays, PNAS (2020).Ģ. The code takes advantage of the equivalence between population coding and stochastic sampling to optimize computations. This model captures effects of set size on the basis of sharing a fixed amount of population activity between items in memory to constrain model parameters it is recommended to fit this model to data from multiple set sizes.
#DOWNIMG MATLAB FULL#
Code to fit a detailed model of population coding: either the original 1D (report dimension only) model with a fixed per-item swap probability, or the full 2D version implementing variability in both cue and report dimensions. Neural Resource model (Stochastic sampling). This MATLAB toolbox brings together models and methods from our lab for analyzing behavioural responses on analogue report (or “delayed estimation”) tasks, where both stimuli and responses are chosen from a continuous parameter space.
